UC Berkeley Center for Targeted Machine Learning and Causal Inference
The Center for Targeted Learning is an interdisciplinary research center for advancing, implementing and disseminating statistical methodology to address problems arising in public health and clinical medicine. We are bringing the rigor and power of classical statistics together with advances in data mining and machine learning to accelerate the development and dissemination of causal inference methods that bring robust insight and evidence to the important public health questions in California and around the world.
Alan Hubbard
Dr. Alan Hubbard is Professor of Biostatistics, Head of the Division of Biostatistics at UC Berkeley, and Head of data analytics core at UC Berkeley SuperFund. His current research interests include causal inference, variable importance analysis, statistical machine learning, estimation of and inference for data-adaptive statistical target parameters, and targeted minimum loss-based estimation. Research in his group is generally motivated by applications to problems in computational biology, epidemiology, and precision medicine.
Maya Petersen
Dr. Maya L. Petersen is an Associate Professor of Biostatistics and Epidemiology at the University of California, Berkeley. Dr. Petersen’s methodological research focuses on the development and application of novel causal inference methods to problems in health, with an emphasis on longitudinal data and adaptive treatment strategies (dynamic regimes), machine learning methods, adaptive designs, and study design and analytic strategies for cluster randomized trials.
Mark van der Laan
Mark Johannes van der Laan is the Jiann-Ping Hsu/Karl E. Peace Professor of Biostatistics and Statistics at the University of California, Berkeley. He has made contributions to survival analysis, semiparametric statistics, multiple testing, and causal inference. He also developed the targeted maximum likelihood methodology. He is a founding editor of the Journal of Causal Inference.
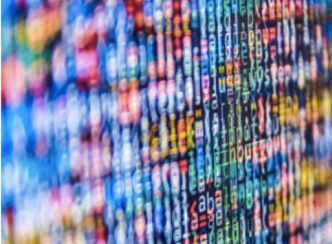
Contact Information
Location
2121 Berkeley Way West
Berkeley , CA 94704
United States